Why I Will Never See an Ensemble Model on an Asset Management Project
- JD Solomon
- Sep 13, 2022
- 4 min read
Updated: Sep 18, 2022
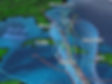
Hurricane tracking is very good these days, especially in forecasting storm intensity and location two to seven days in advance.
Forecasting lifecycle costs (or the total cost of ownership) in asset management programs can learn a lot from forecasting tropical cyclones.
The most powerful model type is the ensemble, but there is little chance we will see it in asset management practice. Here are a few reasons why.
Spaghetti Diagrams
Spaghetti weather diagrams are a simplistic way of quickly conveying a lot of tropical information. Spaghetti diagrams derive their name from the appearance of tens of thin lines, each depicting a separate forecast.
The first lesson for asset managers is that forecast for tropical cyclones includes many lines, whereas the asset management lifecycle forecast includes a single line. There is not much spaghetti in asset management forecasting.
Another lesson is that many different forecasts (and different types of forecasts) are shown on the same diagram. The recognition that there are different types of forecasts underscores that one model is not significant or relevant for all predictions of an uncertain future.
Types of Forecast Models
A model is a system of postulates, data, and inferences presented as a mathematical description of an entity or state of affairs. In math, a model is a bunch of equations.
The term "forecast model" refers to any objective tool used to generate a prediction of a future event, such as the state of the atmosphere. The National Hurricane Center (NHC) uses many models as guidance in the preparation of official track and intensity forecasts.
There are a handful of model types:
1. Statistical models, in contrast, do not explicitly consider the physics of the atmosphere but instead are based on historical relationships between storm behavior and storm-specific details such as location and date.
2. Dynamical models, also known as numerical models, are the most complex and use high-speed computers to solve the physical equations of motion governing the atmosphere.
3. Statistical-dynamical models blend both dynamical and statistical techniques by making a forecast based on established historical relationships between storm behavior and atmospheric variables provided by dynamical models.
4. Trajectory models move a tropical cyclone along based on the prevailing flow obtained from a separate dynamical model.
5. Ensemble (or consensus) models are created by combining the forecasts from a collection of other models.
Accuracy
There were 394 official forecasts issued during the 2021 Atlantic hurricane season, which is above the long-term average number of forecasts and a similar level of activity as the 2016-2018 seasons. The mean National Hurricane Center (NHC) official track forecast errors in the Atlantic basin were close to or below their previous 5-year means. Records for track accuracy were set from 48-72 h in 2021.
The official track forecasts were slightly outperformed by the ensemble (consensus) models at some time periods.
Mean official intensity errors for the Atlantic basin in 2021 were lower than the previous 5-year means at all forecast times. The official forecasts were quite skillful and beat all of the models at 12-36 h and 72 h. Records for intensity accuracy were set from 12-60 h in 2021. Although there is a considerable amount of year-to-year variability, the intensity forecast errors have gradually decreased over the past decade.
While the official intensity forecasts beat the model forecasts in 2021, the top two forecast models were ensemble (consensus) models.
The Power of the Ensemble
It is impossible to definitively predict the future state of uncertain future events, including the atmosphere, because of their chaotic nature. Whether it is observation networks in weather or limited monitoring points in asset management, there is limited resolution in both space and time. Lack of observations introduces uncertainty into the true initial state of the atmosphere.
There are four primary considerations related to ensemble (consensus) forecasts.
1. If the ensembles are close together, the confidence in a forecast is higher. However, the forecast still should be checked against other ensembles (if available) because each ensemble member is part of the analyst’s ideas or preferences.
2. If an ensemble forecast is tightly packed but still diverges from other ensemble (consensus) models, the forecast could be either very arrogant or likely to be correct. This is where forecaster judgment is important.
3. If the ensembles are spread apart, the model has less confidence or the overall forecast is highly uncertain.
4. Ensemble forecasts are appropriate for planning purposes (medium and long-range forecasts) but not applicable for real-time. This is largely a function of the time it takes to collect, process, forecast, and assemble the model.
Asset Management Limitations
Forecasts with ensemble models provide more insights and are usually more accurate than single models. Ensemble forecasts will continue to have limited application in asset management for the following reasons.
It isn't easy to obtain resources for one lifecycle forecast model, much less more than one.
We seldom run things to failure, so judging the forecast results is not practicable.
We seldom perform forecast post-mortems even if the data is available.
We tend to focus more on collecting physical equipment data and less on asset valuation data, which is needed to develop a lifecycle model.
We are usually more focused on the near-term rather than the long term.
What This Means
Forecasting lifecycle costs (or the total cost of ownership) in asset management programs can learn a lot from forecasting tropical cyclones. For several reasons, the ultimate power of forecasting with ensemble models will never be fully utilized in asset management.
But fret not. Ensemble models are used in the black boxes of machine learning to develop business rules!
References
Portions of the article were taken from the NHC Forecast Variation Report for the 2021 Hurricane Season.
JD Solomon Inc provides forecasting services related to asset management and new infrastructure programs. Contact us for more information on our quantitative forecasting services, including those using Monte Carlo simulations.